Avoiding the Great Waste: Connecting Unstructured Data to AI, Analytics via the Cloud
The channel can help enterprises tap the power of their data with cloud-based storage systems.
February 15, 2021
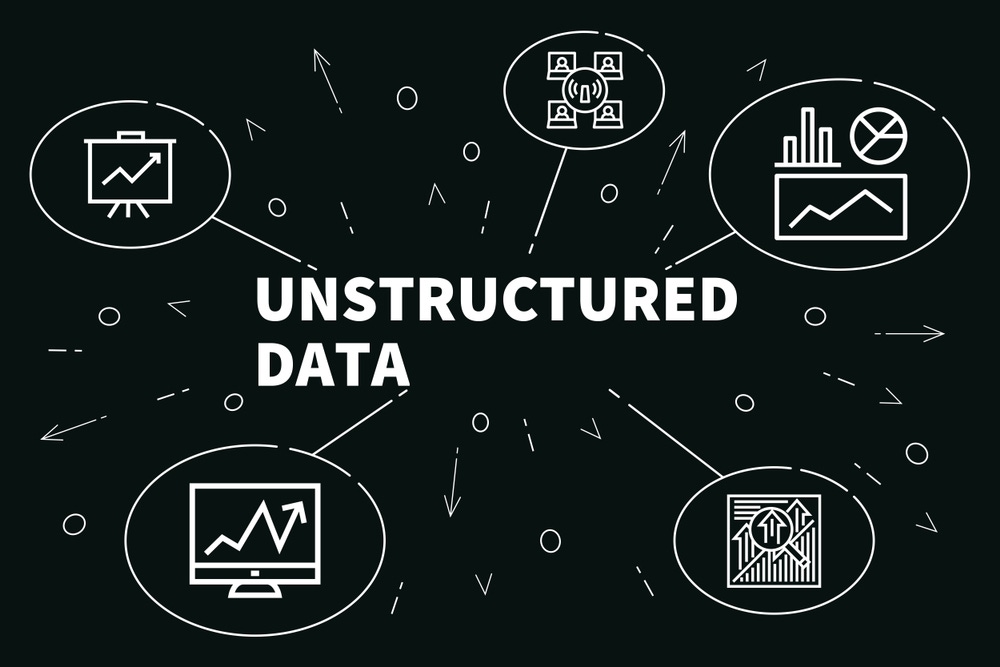
By Russ Kennedy
Russ Kennedy
It’s no secret to IT leaders that business operations can benefit greatly from the incorporation of machine learning (ML) and analytics into day-to-day decision-making. One-third of those leaders say that business analytics is the No. 1 reason they’re interested in adopting machine learning, according to 451 Research. For example, construction firms putting together bids can analyze past proposals to uncover key elements that led to wins and losses. Design firms can quickly identify relevant projects they’ve completed in the past. Compliance officers can use ML to find areas where they are likely to run afoul of regulations in the near future.
But ML can produce these kinds of insights only if it has access to all available data. And right now, that’s an issue. About 80% of enterprise data is unstructured, which includes files, images, emails, CAD files and the like, and typically, unstructured data is stored in discrete silos spread across multiple locations. This state of affairs makes it difficult, at best, for analytics platforms to access it. Unsurprisingly, more than two-thirds of enterprise data goes unused.
Any attempt to analyze a substantial amount of an organization’s unstructured data on-premises will be extremely cumbersome and expensive, assuming it doesn’t fail outright. By using clusters and frameworks such as Hadoop, organizations can deploy analytics and artificial intelligence (AI) on-premises, but the problem of feeding all this scattered, siloed data into the system remains. Additionally, if there are large distances between sites where data is stored and the analytics solutions, latency will slow the process down tremendously.
The channel has a role to play here. If enterprises can get their unstructured data into the cloud, it’s much easier to connect that data to one of the many strong analytics, AI and ML solutions that the large hyperscale cloud providers are already offering to their customers. There’s an opportunity to provide enterprises with cloud-based file storage solutions and strategic guidance that can help them unleash the power of AI and analytics on the vast majority of their data.
AI, ML, Analytics and the Cloud
Data that’s stored in the cloud can be easily connected to sophisticated AI, ML and advanced analytics services, such as Amazon EMR, Amazon Textract, Google BigQuery ML and Azure AI. Whatever type of unstructured data the organization may have — whether it’s video, text or image files — there is almost certainly a cloud service that can derive actionable insights from it, and frequently, these services don’t even require a data scientist. Most employ simple point-and-click interfaces.
Cloud storage is also ideal for applying AI, ML and analytics because it’s built on an object store, which is highly scalable, non-hierarchical and easily accessible. IT can get to the data it needs to access directly, without any need to navigate a structure or a tree. Plus, object stores have a great deal of associated metadata, providing even more information to produce better insights.
Of course, the hard part is moving all that unstructured data from on-premises storage into the cloud, which, for many organizations, could amount to multiple petabytes. Even a fast 1 GB/second upload connection would take four months of continuous transmission to complete a transfer of 10 PB. If time and bandwidth are not in short supply, this may be a good option for some organizations.
But there are other ways to get the data into the cloud faster. Amazon, for instance, has a service called Amazon Snowmobile that will send a tractor trailer to your site, copy up to 100 PB of data into a ruggedized storage container and transport it directly to an AWS cloud data center. Whatever method one uses to transfer the data, the process must be able to understand the …
… original format to be able to read it and then write it to an object store.
However, it’s worth pointing out, if an organization is copying data to the cloud solely for the purpose of connecting that data to analytics, that’s a lot of additional management and cost without gaining any additional benefits. IT must now not only manage file data stored on-premises across multiple locations and systems, but also the copies of this data stored in the cloud, and it needs to be updated, backed up, encrypted and secured.
Thankfully, connecting unstructured data to AI and analytics isn’t the only benefit of moving file data to the cloud. A cloud service can replace traditional on-premises storage with the proper technology deployed.
Hybrid Cloud File Services
Today, there are multiple file data services that operate on a hybrid model: they store the master or “gold” copy of all file data in the cloud, while caching the most frequently used files locally for high-performance. All changes are sent back to the cloud, where they are then propagated back out to local caches to ensure everyone has access to the most recent version of data. In these services, data protection typically takes place automatically in the cloud with low recovery point objectives (RPOs) and recovery time objectives (RTOs), removing another massive headache from IT’s plate.
On-premises analysis of big data and big unstructured data, in particular, is simply no longer necessary given the cloud’s enormous scale and ubiquitous access. The primary challenge is transferring the data to the cloud in the first place and then managing the data in a cost-effective manner. Hybrid-cloud file services enable enterprise IT and their channel partners to simultaneously simplify file data management while making it ready and available for the expanding list of AI, ML services and other analytics.
Russ Kennedy is chief product officer at Nasuni, which provides a file services platform built for the cloud. He previously directed product strategy at Cleversafe through its $1.3 billion acquisition by IBM. Earlier in his career, he served in a variety of product management and development roles, most notably at StorageTek (acquired by Sun Microsystems), where he brought several industry-leading products to market. An avid cyclist and hiker, Kennedy resides in Boulder, Colo., with his family. He has a bachelor’s in computer science from Colorado State University and an MBA in international business from the University of Colorado. You may follow him on LinkedIn or @Nasuni on Twitter.
You May Also Like