The AI Infrastructure Gap
There’s a huge infrastructure gap when it comes to AI, and partners are perfectly positioned to close it.
October 24, 2023
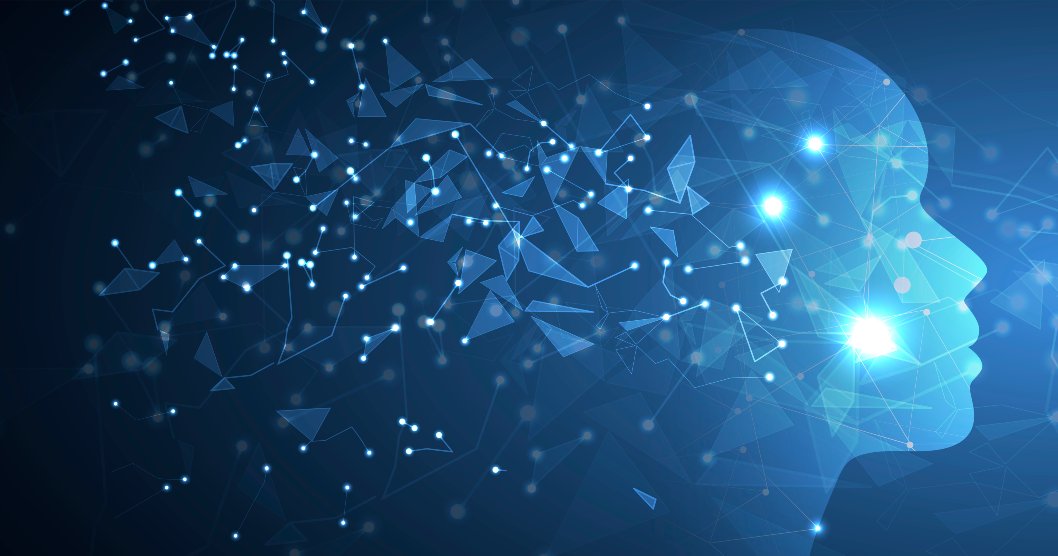
There’s a huge infrastructure gap when it comes to AI, and partners are perfectly positioned to help close it.
Scientific American recently interviewed Alex deVries, whose study of AI energy costs is garnering a great deal of interest — especially his finding that the energy required to fuel the estimated 1.5 million AI server units that will ship per year (from just one vendor) by 2027 will be more than what many small companies use in a year.
In the Scientific American interview, de Vries notes two AI extremes: On the one end, everything is done on AI. In this scenario, every data center will experience effectively a 10-fold increase in energy consumption. On the other end, the growth in demand is completely offset by improving efficiency.
deVries’ suggests that the reality is somewhere in between these two extremes, adding that the demand for AI is likely to grow slowly but surely, and that the time in which it is ramping up will give us enough runway to get ready for it.
This sentiment was shared by Gartner, which recently announced its list of 10 top technology trends. AI, not surprisingly, figured front and center, but Gartner says that the impact of spending on generative AI will not be felt until 2025.
In the meantime, how can partners best prepare their customers to fully exploit AI when the technology is more widely applied?
AI Infrastructure Challenges
In many ways, the challenges that come with a generative AI infrastructure are the same challenges that come with any infrastructure solution — namely, how do we enhance the availability and resilience of systems?
In the case of AI, availability and resilience extends to training and sustained inference.
In a generative AI architecture, the training model refers to the phase in which AI models are trained on extremely large data sets, known as large language models (LLMs). The training model typically includes:
Training infrastructure model
Data storage model
Training algorithms
Optimization and evolution models
The other important piece of a generative AI architecture is the inference model, the phase where trained AI models are used to make predictions or perform tasks in real time for real-world applications. The inference model includes:
Inference infrastructure module
Input data processing
Output presentation
Scalability and performance
To support training and inference models, partners will need to help customers evolve in terms of rack power density, disaster avoidance, and cybersecurity. And, as if that weren’t a big enough challenge, they will need to do so keeping in mind the enormous amount of energy that these systems consume.
“In the worst-case scenario, if we decide we’re going to do everything on AI, then every data center is going to experience effectively a 10-fold increase in energy consumption,” notes deVries in the interview with Scientific American. “That would be a massive explosion in global electricity consumption because data centers, not including cryptocurrency mining, are already responsible for consuming about 1% of global electricity.”
Indeed, partners will need to carefully consider several factors when it comes to AI infrastructure. For example, generative AI requires the accelerated processing performance of power-hungry GPUs. This will increase demand for uninterruptible power supplies and power distribution units (PDUs). The traditional standard of 5kW per rack will soon turn into 20kW per rack.
And, with increased power consumptions comes the need for battery backups to kick in when (not if) power outages occur.
Finally, cooling will be a huge issue. deVries notes in the Scientific American interview that global data centers will add, on average, 50% to energy costs just to keep systems cool.
As high-power-density generative AI systems create more heat than can be dissipated by traditional room cooling systems, customers will increasingly be seeking the help of partners to deploy supplemental cooling solutions.
Eaton Can Help Bridge AI Infrastructure Power Gaps
The infrastructure needed for generative AI systems requires careful design and expertise. Eaton pre-sales support teams are available to help you build these new capabilities and take advantage of incremental revenue and margin opportunities.
This guest blog is part of a Channel Futures sponsorship.
About the Author(s)
You May Also Like